Research Brief: Modeling Harmful Algal Blooms with Machine Learning
1Harmful algal blooms (HABs) are tied to multi-billion dollar losses and transforming aquatic systems. HABs threaten fisheries and ecosystems as sensitive organisms like mussels are threatened by the toxigenic blooms; they can also impact the health of fish, leading to mortalities. Humans may be less likely to consume fish exposed to the blooms, and long-term exposure while fishing presents possible health concerns.
Many freshwater systems have started to experience HABs only recently, though these blooms are often an indicator of more long-term trends. In contrast, some regions have been experiencing blooms for many years, facing annual blooms. The western basin of Lake Erie is known for its large blooms that last throughout the summer.
While HABs can be predicted through nutrient loading, this strategy has been found to be less accurate in extreme bloom years.1 A 2022 study published in Communications Earth & Environment shows that a machine learning approach using observed nutrient loading and large-scale climate indices can improve HAB prediction in Lake Erie.1
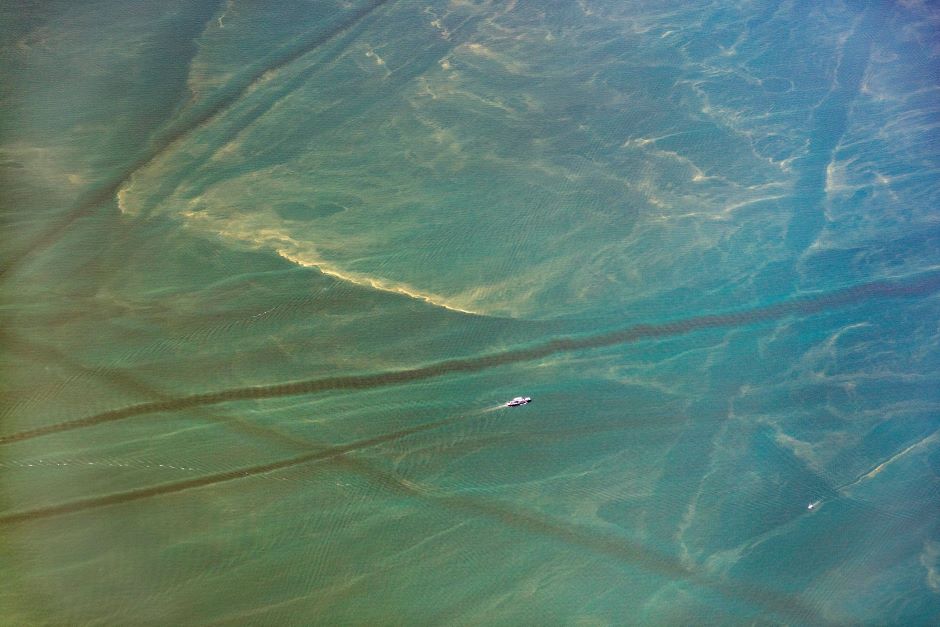
Harmful Algal Bloom in Western Basin of Lake Erie: July 9, 2018 (Credit: Zachary Haslick / Aerial Associates Photography, Inc. via Flickr Public Domain)
Methods
The machine-learning approach builds off of and modifies previous approaches like nutrient monitoring to include a set of physically-based climate indices as potential predictors. A total of eight parameters were considered: total phosphorus, soluble reactive phosphorus, total Kjeldahl nitrogen, total nitrogen, chlorides, total suspended solids, silica dioxide, and sulfate.
The parameters were observed from March to May in order to develop a model input. The model was validated by its ability to predict previous years of extreme blooms. In both 2011 and 2015, the Genetic Algorithm-chemical (GA-chem) model had an overprediction of 1.12 and 7.8 units, respectively. However, this may be a result of unprecedented nutrient loading from the Maumee River. Similar issues arose with the GA-climate (GA-clim) exclusive model.
The models were combined to form what was called the GA-chem-clim model, a model based on chemical loading and climate indices, which was also tested against historical data and events.
Results
Overall, testing the model on previous years’ blooms, the Lake Erie seasonal severity index was successfully predicted using a GA model that used both chemical data and climate indices. The GA-chem-clim model provides a more accurate prediction than the GA-chem or GA-clim exclusive models.
The machine learning approach allows for seasonal predictions to be completed by early June, before the expected peak of HAB activity from July to October. The improved prediction for extreme HABs can provide policymakers ample time to plan for and mitigate any impacts. With over $4.6 billion per annum in estimated damages, HAB prediction strategies must continue to be developed in order to minimize the losses.1
Sources
- Tewari, M., Kishtawal, C.M., Moriarty, V.W. et al. Improved seasonal prediction of harmful algal blooms in Lake Erie using large-scale climate indices. Commun Earth Environ 3, 195 (2022). https://doi.org/10.1038/s43247-022-00510-w
[…] the fishery, there is limited understanding of how exactly climate change will impact the fishery. Modeling and continuous monitoring efforts can help develop a better understanding of conditions and support […]