Research Brief: CyanoHABs Forecasting in Satellite Monitored Lakes
0Lakes everywhere experience harmful algal blooms that can impact water quality, chemical and physical processes, biodiversity, ecosystem health, and environmental services. For this reason, forecasting cyanobacterial blooms is an important future step in resource management.
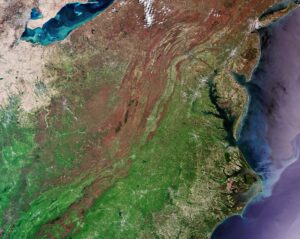
Copernicus Sentinel-3 satellite imagery of the Eastern U.S. (Credit: contains modified Copernicus Sentinel data (2018), processed by ESA, CC BY-SA 3.0 IGO
A 2024 study published in the Journal of Environmental Management sought to create a cyanobacteria forecasting model using satellite lake data.1
The objective of the study was to develop an accurate weekly prediction of cyanobacterial harmful algal blooms (cyanoHABS) across 2192 contiguous United States lakes and reservoirs using satellite data.1
Methods
A Bayesian hierarchical Integrated Nested Laplace Approximation (INLA) model was used to estimate spatiotemporal and predictor effects and forecast cyanobacterial blooms. 2192 satellite-revolved lakes were sampled at a weekly timestamp.
Performance on the INLA model was compared “against support vector classifier and random forest machine learning models; and Dense Neural Network, Long Short-Term Memory (LSTM), Recurrent Neural Network (RNN), and Gneural Network (GNU) neural network models.”1
Predictor variables included surface water temperature, precipitation, mean lake depth, lake surface area, and ambient air temperature and precipitation.
The INLA forecast model was applied to the 2192 lakes in the contiguous United States and was evaluated for accuracy. The forecast sought to predict blooms that met or exceeded the criteria of the World Health Organization’s recreation Alert 1.
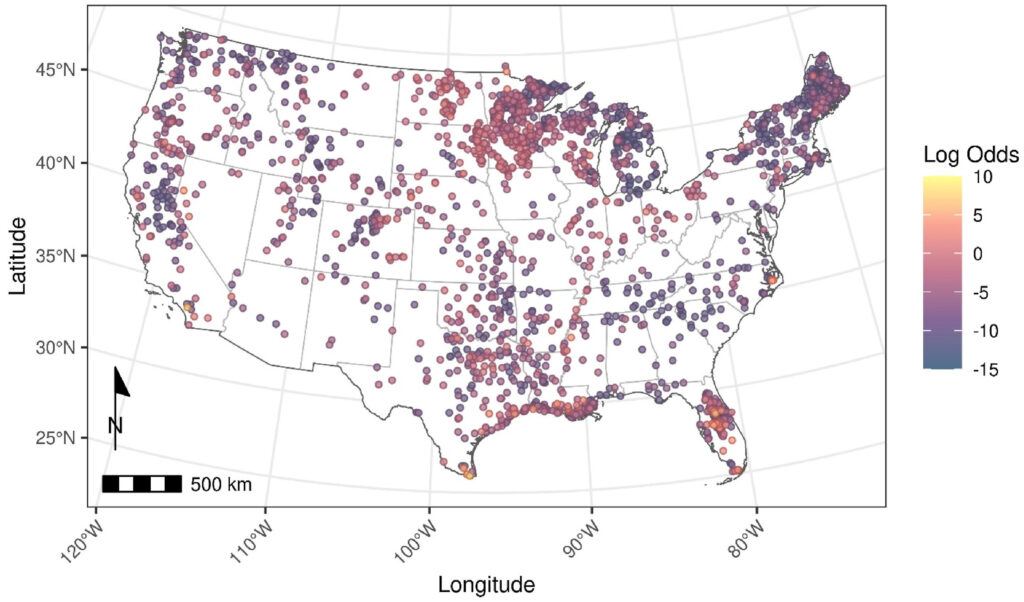
Mean of spatial random effect mapped across lakes. Lakes with relatively higher log-odds of a WHO Alert Level 1 cyanoHAB event are indicated with warm colors, while cool colors represent lakes with relatively lower log-odds of Alert Level 1 cyanoHABs. (Schaeffer et al., 2024)
Results
The “INLA model outperformed the machine learning and neural network models with prediction accuracy of 90% with 88% sensitivity, 91% specificity, and 49% precision.”1
By the conclusion of the study, a reliable INLA model for forecasting cyanoHABs was developed. Out of the 261 weeks in the study period and 432,030 total lake-week observations across all 2192 lakes, 43,482 (10.1 %) lake-weeks were classified as “bloom weeks,” meaning they were above the WHO Alert Level 1 threshold.1
Successful prediction models like INLA are an important tool for resource managers and informing future land use. The INLA model fills a critical gap in cyanoHABs forecasting and builds onto existing monitoring systems and networks that can help improve lake management.
For example, water treatment plants must account for HABs when treating source water for consumption, and the forecasting model can help keep operators informed. However, limitations in precision (resulting from lower bloom prevalence) indicate a need for continued monitoring and sampling of these systems.
Source
- Schaeffer, B. A., Reynolds, N., Ferriby, H., Salls, W., Smith, D., Johnston, J. M., & Myer, M. (2024). Forecasting freshwater cyanobacterial harmful algal blooms for Sentinel-3 satellite resolved U.S. Lakes and reservoirs. Journal of Environmental Management, 349, 119518. https://doi.org/10.1016/j.jenvman.2023.119518